Expansive linguistic representations to predict interpretable odor mixture discriminability
해석 가능한 냄새 혼합물 구별 가능성을 예측하기 위한 광범위한 언어 표현
Introduction
1. Previous Studies
1) Monomolecular에 대한 후각 연구: 단분자
- chemoinformatic structural descriptor (화학 정보, 구조에 관한 설명자 활용)
- semantic descriptors (사람이 해석할 수 있는 의미론적 설명자 활용)
2) Mixtures에 대한 후각 연구: 혼합물
- chemoinformatic structural descriptor (화학 정보, 구조에 관한 설명자 활용)
⇒ Semantic descriptors로 Mixture 간의 유사도 및 구별 가능성을 예측
2. Two Solutions to predict the results of a discriminability test
1. 예측하고자 하는 대상
: Discrimination test의 결과 (피실험자가 올바르게 답한 비율)
※ Discrimination test
2. 해결 방안
1) Direct Model (기존 논문)
- chemoinformatic descriptors를 사용해 각 혼합물에 대해 화학적 feature로 벡터 추출
- 추출한 2개의 벡터 사이의 각 거리를 계산해 Discrmination test 결과 예측
2) Semantic Model (해당 논문)
- semantic descriptors를 사용해 각 혼합물에 대해 21개의 선택된 semantic attributes feature로 Metric 추출
- Metric Learning을 사용해 학습 후 매핑된 Discrmination test 결과로 예측
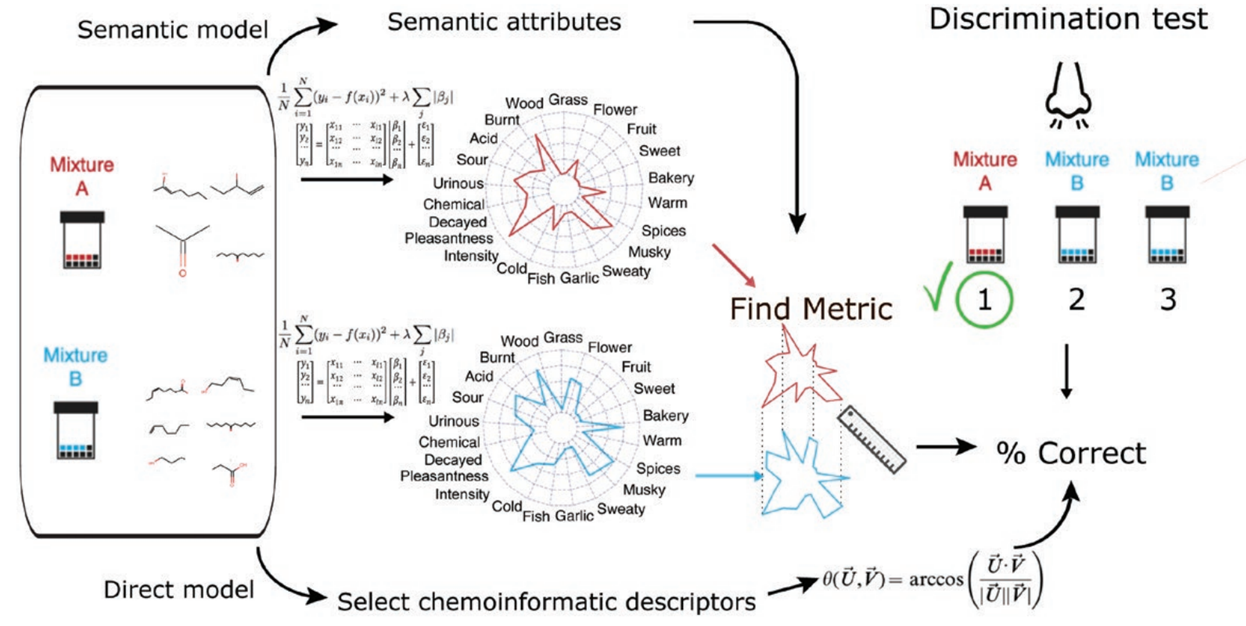
Methods
1. The overall structure of SEMANTIC MODEL
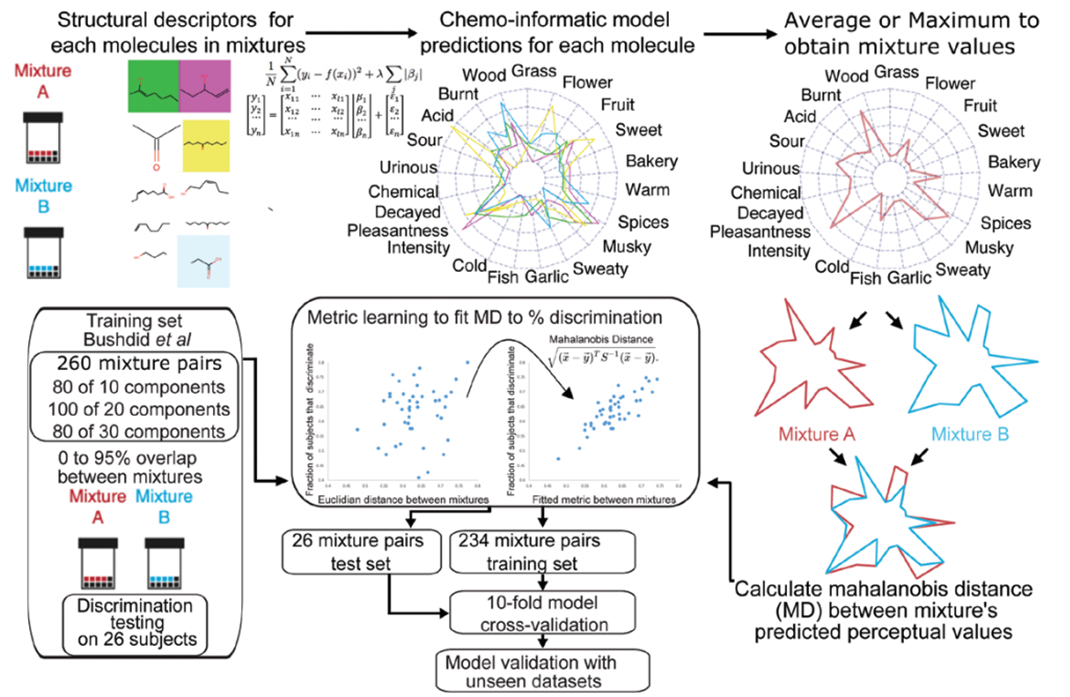
2. Specific Steps
1) Structural descriptors for each molecules in mixtures
: 혼합물 내의 구조적 속성에 관한 분자 결정 by Dragon descriptors 사용
2) Chemo-informatic model predictions for each molecule
: 결정한 분자에 대해 단분자의 21개의 semantic descriptor feature 예측
→ 아래의 연구에서 구현한 모델 사용
3) Average or Maximum to obtain mixture values
: 단분자 semantic descriptor feature를 평균 혹은 최댓값으로 혼합물의 semantic feature 사용
4) Metric Learning to fit MD to % discrimination
: 혼합물의 semantic feature vector를 혼합물 구별 가능성 결과 값을 목표로 Metric 학습
- Feature definition
: 혼합물 A와 B의 i번째 feature 차의 제곱
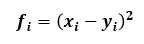
- Lasso Model Training
- feature vector와 사람의 혼합물 구별 가능성 매핑하며 학습
(Training dataset : Bushdid et al, 2024로 260개의 혼합물 쌍)
- Lasso linear regression : 유의미하지 않는 변수들에 대한 가중치를 0에 가깝게
→ 최소한의 설명자로 중복된 descriptors 제거 (21개 중 10개 제거)
ex. wood와 grass의 semantic descriptor는 유사하므로, 둘 중 하나인 grass
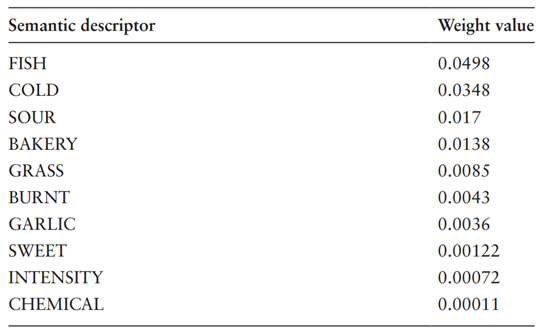
- Mahalanobis distance metric
: 주변의 점들의 상대적 위치를 고려하여 나타낸 거리
→ 거리가 짧을수록 두 개의 혼합물 유사
1) Mahalanobis distance로 두 냄새 혼합물 간의 거리 측정
2) 모델의 출력 값 (판별 가능성 예측 값)을 [0, 1] 구간으로 맞추는 데 사용
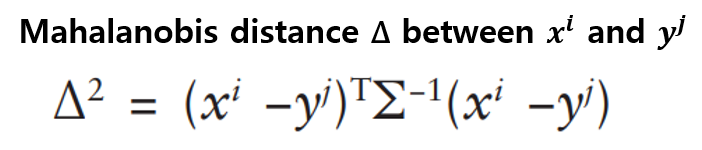
Results
1. RMSE of the predictions of the Semantic model
2. Comparison of the predicted discriminability
3. Languages as a measure of smell
4. Metric-derived properties of the olfactory space
5. Density plot representing the 548 predictions (Learned metric) of the Semantic model for the 3 datasets against the experimental results for discriminability
6. Diagram of the different possibilities of interpretation of the psychometric perceptual curves relative to the existence of olfactory metamers
'예바의 LAB' 카테고리의 다른 글
[논문 발표] Expansive linguistic representations to predict interpretable odor mixture discriminability (2) | 2024.07.05 |
---|---|
[논문 발표] SRDFM (2) | 2024.07.05 |
LINUX(리눅스) 명령어 (4) | 2024.04.15 |
[논문 리뷰] SRDFM (2) (0) | 2024.04.14 |
[논문 리뷰] SRDFM (1) (4) | 2024.04.06 |